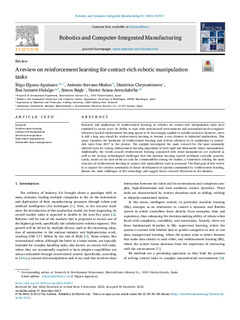
Izenburua
A review on reinforcement learning for contact-rich robotic manipulation tasksBeste instituzio
Electrotecnica Alavesa S.L.Aalborg Universitet (Denmark)
Montajes Mantenimiento y Automatismos Eléctricos Navarra
Bertsioa
Bertsio argitaratua
Eskubideak
© 2023 The AuthorsSarbidea
Sarbide irekiaArgitaratzailearen bertsioa
https://doi.org/10.1016/j.rcim.2022.102517Non argitaratua
Robotics and Computer-Integrated Manufacturing Vol. 81. N. artículo 102517Argitaratzailea
ElsevierGako-hitzak
Reinforcement learning
Contact-rich manipulation
Industrial manipulators
Rigid object manipulation ... [+]
Contact-rich manipulation
Industrial manipulators
Rigid object manipulation ... [+]
Reinforcement learning
Contact-rich manipulation
Industrial manipulators
Rigid object manipulation
Deformable object manipulation [-]
Contact-rich manipulation
Industrial manipulators
Rigid object manipulation
Deformable object manipulation [-]
Laburpena
Research and application of reinforcement learning in robotics for contact-rich manipulation tasks have exploded in recent years. Its ability to cope with unstructured environments and accomplish hard ... [+]
Research and application of reinforcement learning in robotics for contact-rich manipulation tasks have exploded in recent years. Its ability to cope with unstructured environments and accomplish hard-to-engineer behaviors has led reinforcement learning agents to be increasingly applied in real-life scenarios. However, there is still a long way ahead for reinforcement learning to become a core element in industrial applications. This paper examines the landscape of reinforcement learning and reviews advances in its application in contact-rich tasks from 2017 to the present. The analysis investigates the main research for the most commonly selected tasks for testing reinforcement learning algorithms in both rigid and deformable object manipulation. Additionally, the trends around reinforcement learning associated with serial manipulators are explored as well as the various technological challenges that this machine learning control technique currently presents. Lastly, based on the state-of-the-art and the commonalities among the studies, a framework relating the main concepts of reinforcement learning in contact-rich manipulation tasks is proposed. The final goal of this review is to support the robotics community in future development of systems commanded by reinforcement learning, discuss the main challenges of this technology and suggest future research directions in the domain. [-]
Sponsorship
Comisión EuropeaProjectu ID
info:eu-repo/grantAgreement/EC/H2020/8570617/EU/Networking for research and development of human interactive and sensitive robotics taking advantage of additive manufacturing/R2P2Bildumak
Item honek honako baimen-fitxategi hauek dauzka asoziatuta:
Bestelakorik adierazi ezean, itemaren baimena horrela deskribatzen da: Attribution-NonCommercial-NoDerivatives 4.0 International
Related items
Showing items related by title, author, creator and subject.
-
Neural Network Direct Control with Online Learning for Shape Memory Alloy Manipulators
Loidi Eguren, Ion (MDPI AG, 2019)New actuators and materials are constantly incorporated into industrial processes, and additional challenges are posed by their complex behavior. Nonlinear hysteresis is commonly found in shape memory alloys, and the ... -
Optimizing Polymer Lab-on-Chip Platforms for Ultrasonic Manipulation: Influence of the Substrate
Martin-Mayor, Alain; Bou-Ali, M. Mounir (MDPI, 2015)The choice of substrate material in a chip that combines ultrasound with microfluidics for handling biological and synthetic microparticles can have a profound effect on the performance of the device. This is due to the ... -
A review on reinforcement learning for contact-rich robotic manipulation tasks
Serrano Muñoz, Antonio; Chrysostomou, Dimitrios; Inziarte Hidalgo, Ibai; Bogh, Simon; Arana Arexolaleiba, Nestor; Electrotecnica Alavesa S.L.; Mondragon Goi Eskola Politeknikoa; Aalborg University; Montajes Mantenimiento y Automatismos Eléctricos Navarra