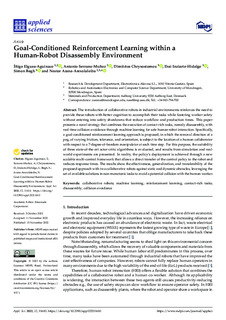
Izenburua
Goal-Conditioned Reinforcement Learning within a Human-Robot Disassembly EnvironmentEgilea
Egilea (beste erakunde batekoa)
Beste instituzio
Aalborg Universitet (Denmark)Electrotecnica Alavesa S.L.
Bertsioa
Bertsio argitaratua
Eskubideak
© 2022 The AuthorsSarbidea
Sarbide irekiaArgitaratzailearen bertsioa
https://doi.org/10.3390/app122211610Non argitaratua
Applied Sciences Vol. 12. Nº 22. Article 11610. November, 2022Argitaratzailea
MDPIGako-hitzak
collaborative robots
machine learning
reinforcement learning
contact-rich tasks ... [+]
machine learning
reinforcement learning
contact-rich tasks ... [+]
collaborative robots
machine learning
reinforcement learning
contact-rich tasks
Disassembly
collision avoidance [-]
machine learning
reinforcement learning
contact-rich tasks
Disassembly
collision avoidance [-]
Laburpena
The introduction of collaborative robots in industrial environments reinforces the need to provide these robots with better cognition to accomplish their tasks while fostering worker safety without en ... [+]
The introduction of collaborative robots in industrial environments reinforces the need to provide these robots with better cognition to accomplish their tasks while fostering worker safety without entering into safety shutdowns that reduce workflow and production times. This paper presents a novel strategy that combines the execution of contact-rich tasks, namely disassembly, with real-time collision avoidance through machine learning for safe human-robot interaction. Specifically, a goal-conditioned reinforcement learning approach is proposed, in which the removal direction of a peg, of varying friction, tolerance, and orientation, is subject to the location of a human collaborator with respect to a 7-degree-of-freedom manipulator at each time step. For this purpose, the suitability of three state-of-the-art actor-critic algorithms is evaluated, and results from simulation and real-world experiments are presented. In reality, the policy’s deployment is achieved through a new scalable multi-control framework that allows a direct transfer of the control policy to the robot and reduces response times. The results show the effectiveness, generalization, and transferability of the proposed approach with two collaborative robots against static and dynamic obstacles, leveraging the set of available solutions in non-monotonic tasks to avoid a potential collision with the human worker. [-]
Sponsorship
Comisión EuropeaProjectu ID
info:eu-repo/grantAgreement/EC/H2020-ECSEL/no 876852/EU/Verification and Validation of Automated Systems’ Safety and Security/VALU3SBildumak
Item honek honako baimen-fitxategi hauek dauzka asoziatuta: