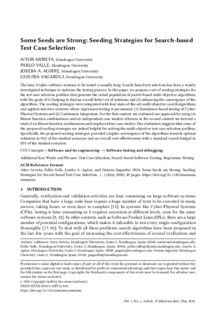
Izenburua
Some Seeds are Strong : Seeding Strategies for Search-based Test Case SelectionBertsioa
Postprinta
Eskubideak
© 2022 Association for Computing MachinerySarbidea
Sarbide irekiaArgitaratzailearen bertsioa
https://doi.org/10.1145/3532182Non argitaratua
ACM Transactions on Software Engineering and Methodology. Argitaratzailea
ACMGako-hitzak
Test Case SelectionSearch-based Software Testing
Regression Testing
Laburpena
The time it takes software systems to be tested is usually long. Search-based test selection has been a widely investigated technique to optimize the testing process. In this paper, we propose a set o ... [+]
The time it takes software systems to be tested is usually long. Search-based test selection has been a widely investigated technique to optimize the testing process. In this paper, we propose a set of seeding strategies for the test case selection problem that generate the initial population of pareto-based multi-objective algorithms, with the goals of (1) helping to find an overall better set of solutions and (2) enhancing the convergence of the algorithms. The seeding strategies were integrated with four state-of-the-art multi-objective search algorithms and applied into two contexts where regression-testing is paramount: (1) Simulation-based testing of Cyber-Physical Systems and (2) Continuous Integration. For the first context, we evaluated our approach by using six fitness function combinations and six independent case studies, whereas in the second context we derived a total of six fitness function combinations and employed four case studies. Our evaluation suggests that some of the proposed seeding strategies are indeed helpful for solving the multi-objective test case selection problem. Specifically, the proposed seeding strategies provided a higher convergence of the algorithms towards optimal solutions in 96% of the studied scenarios and an overall cost-effectiveness with a standard search budget in 85% of the studied scenarios. [-]