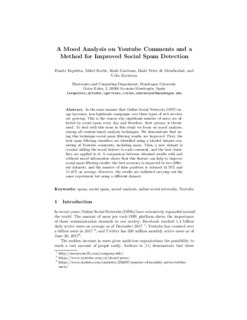
Título
A Mood Analysis on Youtube Comments and a Method for Improved Social Spam DetectionVersión
Postprint
Derechos
© Springer International Publishing AG, part of Springer Nature 2018. This is a post-peer-review, pre-copyedit version of an article published in Hybrid Artificial Intelligent Systems. HAIS 2018. Lecture Notes in Computer Science, vol 10870. The final authenticated version is available online at: https://doi.org/10.1007/978-3-319-92639-1_43Acceso
Acceso embargadoVersión del editor
https://doi.org/10.1007/978-3-319-92639-1_43Publicado en
Hybrid Artificial Intelligent Systems (HAIS 2018). Pp. 514-525. Lecture Notes in Computer Science Vol.10870. Springer,Editor
SpringerPalabras clave
spam
social spam
mood analysis
online social networks ... [+]
social spam
mood analysis
online social networks ... [+]
spam
social spam
mood analysis
online social networks
Youtube [-]
social spam
mood analysis
online social networks
Youtube [-]
Resumen
In the same manner that Online Social Networks (OSN) usage increases, non-legitimate campaigns over these types of web services are growing. This is the reason why signi cant number of users are affec ... [+]
In the same manner that Online Social Networks (OSN) usage increases, non-legitimate campaigns over these types of web services are growing. This is the reason why signi cant number of users are affected by social spam every day and therefore, their privacy is threatened. To deal with this issue in this study we focus on mood analysis, among all content-based analysis techniques. We demonstrate that using this technique social spam filtering results are improved. First, the best spam filtering classifiers are identified using a labeled dataset consisting of Youtube comments, including spam. Then, a new dataset is created adding the mood feature to each comment, and the best classifiers are applied to it. A comparison between obtained results with and without mood information shows that this feature can help to improve social spam filtering results: the best accuracy is improved in two different datasets, and the number of false positives is reduced 13.76% and 11.41% on average. Moreover, the results are validated carrying out the same experiment but using a different dataset. [-]
Sponsorship
Gobierno de EspañaID Proyecto
GE/Programa Estatal de Investigacion, Desarrollo e Innovación orientada a los retos de la sociedad en el marco del Plan Estatal de Investigación Científica y Técnica y de Innovación 2013-2016, convocatoria del 2017/TIN2017-84658-C2-2-R/Integración de Conocimiento Semántico para el Filtrado de Spam basado en Contenido/SKI4SPAMColecciones
- Congresos - Ingeniería [423]